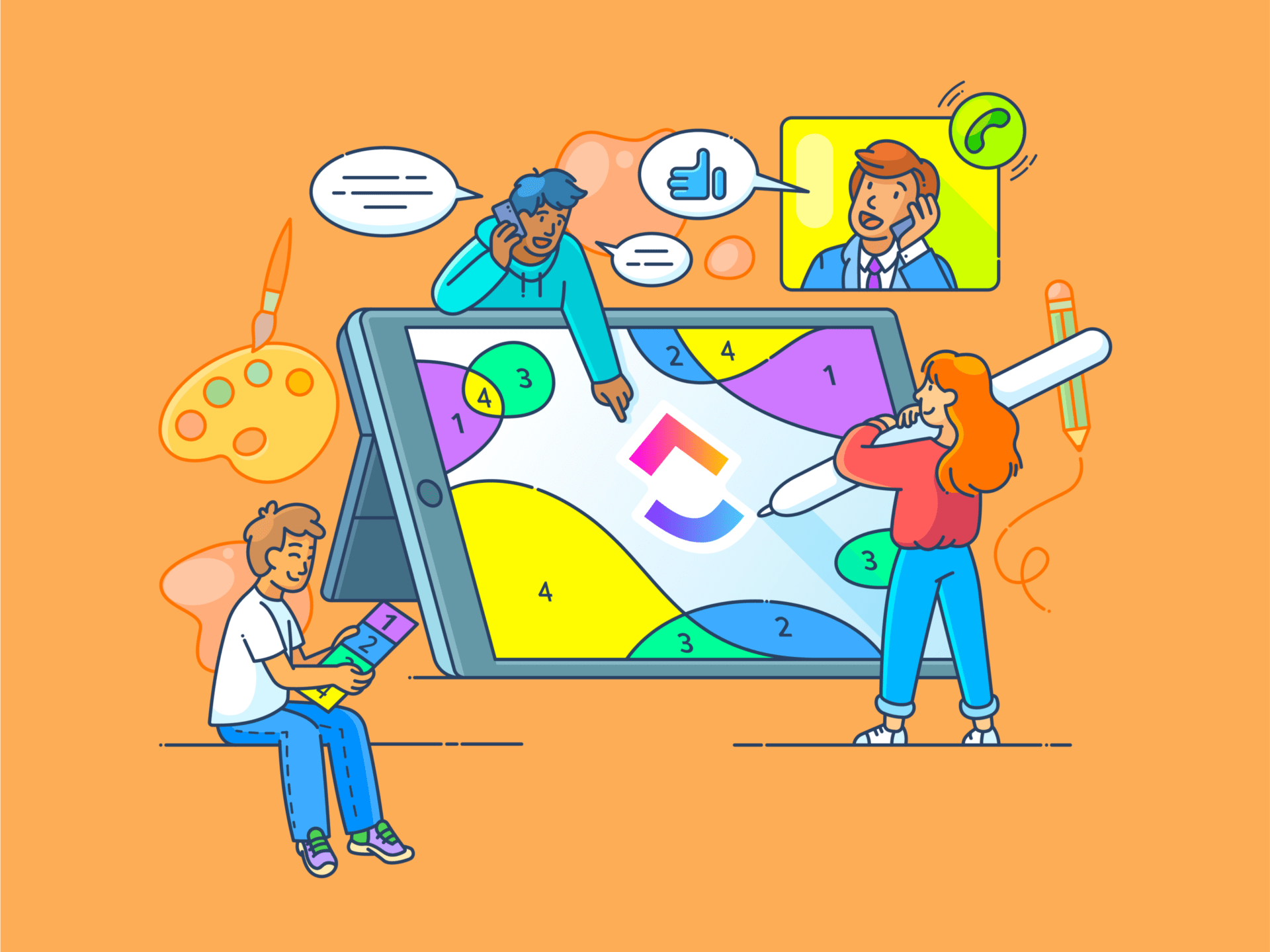
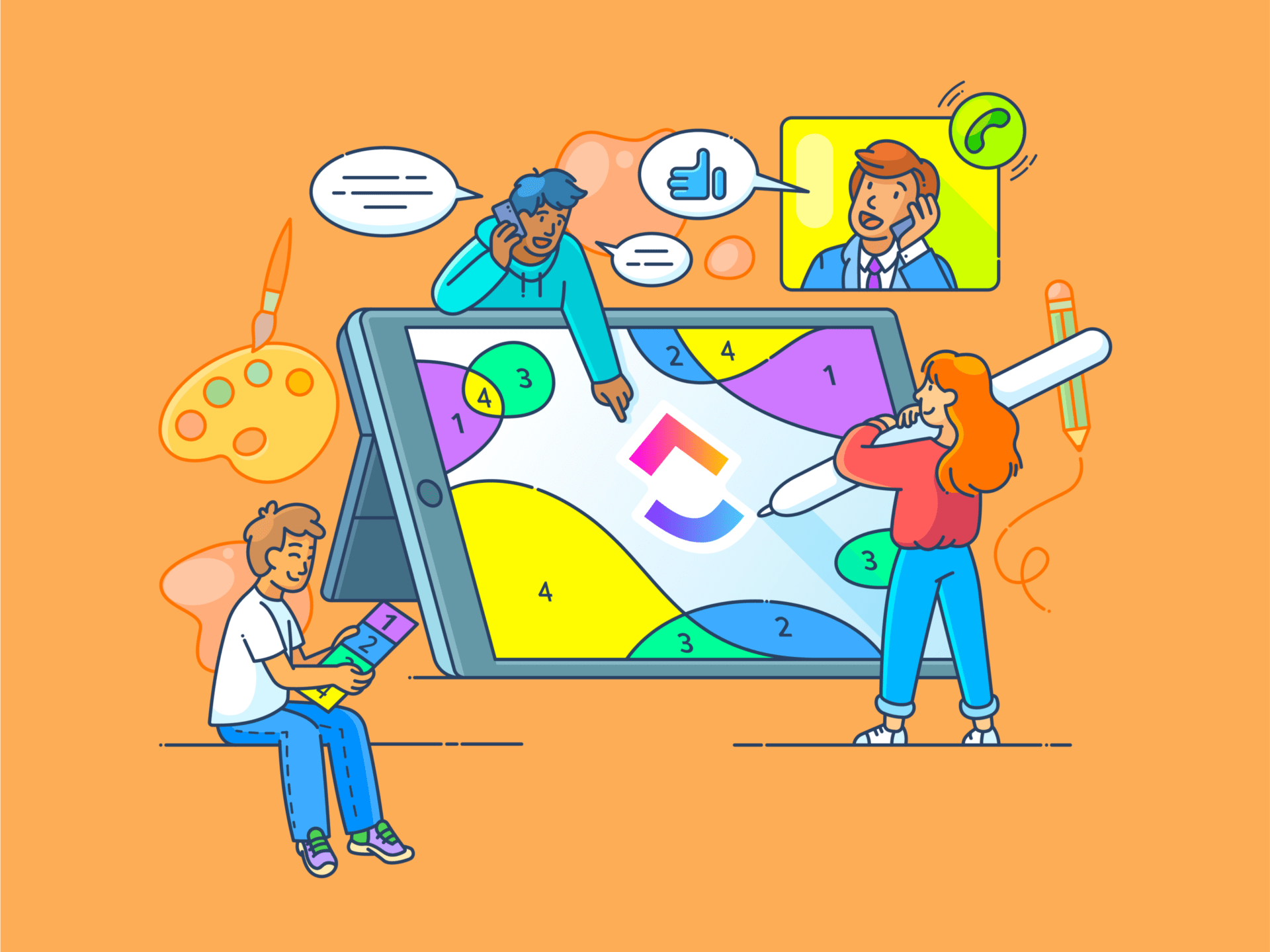
The Framingham Heart Study is an ongoing, long-term study that aims to understand the risk factors for heart disease. By controlling for variables such as age, gender, and lifestyle factors like smoking and diet, researchers aim to isolate the effects of specific risk factors.
The study has already led to groundbreaking findings about cholesterol and blood pressure. 🩺
Control variables—age, gender, and so on in the example above—are crucial for obtaining meaningful results in any research endeavor. By keeping certain elements constant, researchers ensure their findings reflect the true impact of the variable studied.
This article will explore why control variables matter and how they can be managed effectively to achieve credible research results.🔍
- What Are Control Variables?
- How to Identify Control Variables in Research
- Examples of Control Variables in Different Fields
- How to Set up Control Variables in an Experiment
- Common Mistakes When Handling Control Variables
- Control Variables vs. Confounding Variables
- Best Practices for Using Control Variables
- Elevate Your Research with ClickUp
What Are Control Variables?
Ever wondered how researchers cut through the noise to find clear, actionable insights? That’s where control variables come in. 💡
A control variable is a specific factor intentionally kept constant during an experiment to prevent it from influencing the outcome. Although these variables are not the primary focus of the study, their management is essential for ensuring that the results are attributable to the independent variable being tested.
Control variables vary widely depending on the research context, including demographic factors, environmental conditions, or methodological details. Identifying appropriate control variables is a crucial step in the research design process, as it helps establish a clear framework for the study.
Examples of control variables:
Demographics: Age, gender, or income in sociological studies
Environmental conditions: Temperature, light, or humidity in lab experiments
Methodological details: Equipment type or time of data collection in clinical research
Why are control variables important?
Imagine baking a cake. You wouldn’t change the oven temperature randomly or skip the baking powder, right? The same principle applies to research. If control variables aren’t managed, your “recipe” for accurate results falls apart.
In research, independent and dependent control variables must be considered. Independent variables are the factors that researchers alter to observe their effects. Meanwhile, dependent variables are the outcomes measured in response to changes in the independent variables.
Unlike these two, control variables may not be the primary focus of a study. However, they are crucial for drawing clear and valid conclusions about the relationships between your independent and dependent variables. By minimizing external influences, control variables help ensure that your results are truly due to the factor you are testing.
📌 For instance, in medical research, controlling variables such as age, diet, and exercise is essential when testing a new drug. Without these controls, it becomes difficult to determine if health changes result from the drug or other lifestyle factors. Control variables ensure that the observed results are directly related to the treatment rather than confounded by unrelated influences.
Control variables also enhance the replicability of your research. Other researchers conducting the same experiment using the same control variables should obtain similar results. This strengthens the reliability of your findings.
Essentially, the control variable is the foundation of a well-designed experiment. It reduces noise, clarifies relationships, and protects the validity of your research, allowing you to derive confident, data-driven conclusions.
How to Identify Control Variables in Research
Think of identifying a control variable as detective work. Your job is to spot the factors that might sneak in and mess with your results. Here’s how you go about it.
Step 1: Define your independent and dependent variables
Start with a clear project plan in place. Your first step is clearly defining what you’re testing (the independent variable) and what you’re measuring (the dependent variable).
For example, if you’re examining how different fertilizers affect plant growth, the fertilizer type is your independent variable, and plant growth is the dependent variable.
Step 2: Identify potential influences
Think about all the factors that could impact your results. In the fertilizer experiment, factors such as sunlight, water, soil type, and temperature could all influence plant growth. These are potential control variables—factors that need to stay consistent.
Step 3: Decide which variables to control
Once you’ve identified the potential influences, determine which ones you can realistically control.
For example, you can control the amount of water and sunlight each plant receives, but factors like natural fluctuations in humidity might be harder to regulate.
💡 Pro Tip: Before conducting your experiment, run a trial to check that each control variable is stable. This helps you catch any unforeseen issues.
Examples of Control Variables in Different Fields
Control variables are vital in both experimental and non-experimental research.
In an experiment testing an experimental treatment, researchers must analyze data while keeping certain variables constant, such as participant variables like age and marital status. By tracking predictor variables, they can better understand how these factors influence the treatment’s effectiveness, ensuring the results reflect the true impact of the treatment rather than external influences.
Let’s examine examples from different fields to see how they contribute.
Environmental studies
Consider a study examining the impact of ocean acidity on coral bleaching. The acidity level of seawater (measured in pH) is the independent variable; the degree of bleaching observed in the corals is the dependent variable. The control variables here would be factors like temperature (varying between seasons), light exposure, and ocean pollution from various sources, such as nutrient runoff and microplastics.
Psychology
The main consideration in psychological research is the need to control participant variability. For example, if you’re studying whether caffeine improves memory recall, other variables like participant age, test environment, and type of memory test used should be controlled. This helps isolate the effects of caffeine, reducing the likelihood of other factors affecting the results.
Medicine
For medical research like testing whether a vitamin D supplement improves alertness, extraneous variables like participants’ diet, screen time, and caffeine intake must be controlled. This ensures that any changes in alertness are attributable to the supplement itself.
Economics
In non-experimental research, a control variable helps interpret complex data. For example, variables like age, marital status, and health need to be controlled when studying the relationship between income and happiness. Without doing so, the results might reflect influences from these unrelated factors, making it difficult to draw accurate conclusions.
Also Read: How to Conduct a Customer Behavior Analysis
Mechanical engineering
Control variables are essential when testing material strength. For example, if you are studying how materials perform under stress, environmental conditions like humidity, temperature, and pressure should be kept constant to ensure the accuracy of the results.
💡 Pro Tip: Documenting control variables in advance helps avoid inconsistencies during the experiment and ensures reliable outcomes.
How to Set up Control Variables in an Experiment
If control variables aren’t kept strictly in check, they can easily introduce bias or inconsistencies. Using tools to track and manage all aspects of your research can ensure thorough documentation of your control variables. One such tool is ClickUp—find out how.
Documenting control variables with ClickUp
Recording control variables accurately is non-negotiable for reliable results. ClickUp Table View allows you to organize and track these variables easily.
Creating a table listing all the controlled variables lets you ensure everyone knows which conditions must remain constant.
Imagine having a detailed table where each row represents a specific control variable—like temperature or pressure—and columns track critical details like team members assigned, current conditions, and required updates. With ClickUp, this level of organization is easy to achieve.
How it works:
Centralized Tracking: Create a table listing all control variables. For example, a researcher studying plant growth might list variables like sunlight exposure, water levels, and soil type.
Custom Fields for Precision: Use ClickUp Custom Fields to add layers of detail, such as numerical values, time intervals, or status updates. This gives you real-time insights into your control variables and their current state.
Using ClickUp Custom Fields lets you measure and compare the results across multiple tests to validate your findings and understand how replicable your test is.
📌 Example: In a long-term medical trial, you can use Custom Fields to log exact dosage times, participant conditions, and environmental factors, ensuring nothing is missed.
Here’s what a customer says about how ClickUp’s features support their workflow:
Creating detailed task lists and tracking progress
ClickUp doesn’t just help you document variables—it keeps you on top of the entire experiment. By creating detailed task lists, you can ensure every step is accounted for and track progress as you go.
How researchers benefit:
- Set tasks for each variable: Assign tasks to team members to monitor and manage specific variables
- Track progress with status updates: Use customizable statuses like “In Progress,” “Under Review,” or “Complete” to see where each task stands at a glance
- Collaborate seamlessly: Use comments and mentions to discuss updates or flag issues directly within each task
💡 Pro Tip: Break your experiment into smaller milestones. Assign deadlines for each phase and track real-time progress with ClickUp’s Gantt Chart View.
Applying formulas to manage control variables
For more advanced project tracking, you can apply formulas within the table to automate calculations. For example, you can calculate the temperature range across different trials or track the total time a controlled variable has been maintained.
Here are a few formulas that can be used to manage control variables:
- Use the DATE function to calculate time differences between variable changes and track consistency
- Apply the DAYS function to calculate the difference between when a control variable was set and when it was adjusted, ensuring no deviations go unnoticed
Formulas like these help researchers maintain control and precision in projects that manual tracking can’t match.
Managing control variables with reminders
Consistency is key, especially when experiments run over extended periods. ClickUp Reminders allows you to set regular notifications to ensure control variables are checked and recorded on time. You can set up reminders for recalibrating equipment or checking environmental factors like humidity or light levels.
You can also assign these reminders to specific team members. Use project management control charts to help you stay on track throughout the experiment.
Common Mistakes When Handling Control Variables
A high school student sets out to win their science fair by testing different fertilizers on plant growth. But despite their best efforts, the results are all over the place. Why? They forgot to keep factors like sunlight and water consistent. This simple oversight shows how important control variables are—not just for school projects but for every research project.
Even seasoned researchers can slip up when managing control variables, leading to inaccurate results or unreliable conclusions. Avoiding these common pitfalls is crucial.
Mistake #1: Overlooking important variables
One of the most frequent mistakes is not identifying all relevant control variables. Researchers may be so focused on the independent and dependent variables that they fail to consider external factors that could influence the results.
For example, ignoring other drugs that patients may be consuming could skew the outcomes when testing a new drug.
How to avoid it:
Before starting your experiment, consult with your colleagues to brainstorm every possible factor affecting your results. Conduct thorough research before beginning your experiment. Record all the variables you need to consider in your Standard Operating Procedure (SOP) using tools like ClickUp Docs to keep everything organized.
Mistake #2: Failing to monitor variables consistently
It’s not enough to identify control variables; they must be monitored throughout the experiment.
For example, if you’re testing how light affects plant growth, you must regularly check that all plants receive the same amount of light. Without continuous monitoring, minor variations could cause significant changes in your results.
How to avoid it:
- Use tools like ClickUp Reminders to set regular notifications for checks and recalibrations
- Assign monitoring responsibilities to specific team members and track progress in ClickUp’s Task View
- Regularly log changes or updates to ensure consistency across the board
💡 Pro Tip: Automate where you can! For instance, use ClickUp’s Brain feature to track and flag real-time changes so nothing slips through the cracks.
Mistake #3: Inconsistent documentation
Another common mistake is poor documentation. Even if your control variables are well managed, failing to maintain proper documentation can create confusion later on. Researchers may struggle to replicate results or even remember precisely what conditions were maintained.
How to avoid it:
- Create detailed logs in ClickUp’s Table View, listing all control variables, their current status, and any changes over time
- Use Custom Fields to add critical details like numerical values, time intervals, and team member responsibilities
- Regularly review and update your documentation to ensure it stays accurate
💡 Pro Tip: Think of documentation as your experiment’s safety net. The more detailed it is, the easier it’ll be to replicate your findings—and impress your peers!
Control Variables vs. Confounding Variables
Although control and confounding variables play crucial roles in research, they serve different purposes. Understanding the difference between these two concepts can help you design more robust experiments and ensure the accuracy of your results. So far, we have seen what control variables are. Now, let’s understand confounding variables.
What are confounding variables?
Confounding variables sneak into your experiment unnoticed, affecting both the independent and dependent variables. These variables create confusion by suggesting that the observed effect may be due to something other than the variable you intended to study.
For example, if you’re studying the effects of a new teaching method on student performance but fail to account for students’ pre-existing knowledge, that pre-existing knowledge becomes a confounding variable, skewing your results.
Why do confounding variables matter?
Confounding variables can invalidate your experiment’s findings. If you don’t control for these variables, your data may show a correlation that doesn’t exist. This is a significant issue in fields like medical research, where confounding variables can make a new treatment seem more or less effective than it truly is.
For instance, a drug might appear effective when, in reality, participants’ diets or exercise routines are driving the results.
How to control for confounding variables
There are several strategies researchers can use to mitigate the influence of confounding variables:
- Randomization: Randomly assign participants to an experimental and a control group to distribute potential confounding factors evenly. This helps balance out variables like age or health conditions that might otherwise distort your results
- Matching: Pair participants based on confounding factors. For example, in psychological research, participants can be paired by age or cognitive ability to control for these confounders
- Statistical control: Statistical methods, such as regression analysis, can be used to account for confounding variables after data collection. This allows you to isolate the effects of the independent variable while accounting for confounders that may have been missed
💡Pro Tip: When designing your experiment, list potential confounding variables and consider how to minimize their impact through careful experiment design or statistical control.
Best Practices for Using Control Variables
Effectively managing control variables ensures that your experimental results are reliable and free from external influences. Following these industry-standard best practices can help you maintain consistency and accuracy in your research.
Identify all relevant control variables
Before conducting any experiment, thoroughly identify all the variables that need to remain constant. Failing to account for certain environmental or procedural factors can lead to inaccurate results. Depending on your field of research, consider factors like temperature, humidity, and participant behavior. This step is crucial for experiments in biological sciences, social sciences, and clinical trials, where minor external influences can significantly affect the outcome.
Standardize your methods
Ensure that the experimental procedure involving control variables is standardized across all experimental trials. This means creating uniform protocols, such as using the same equipment, administering tests simultaneously, and maintaining consistent lab conditions. This prevents any variability that could interfere with the dependent variable.
ClickUp Docs is perfect for creating a Standard Operating Procedure (SOP) that outlines every step of your experiment and ensures that all control variables are accounted for across trials.
Use ClickUp Docs to maintain an easily accessible and shareable log of your control variables. By integrating ClickUp Custom Fields into your documentation, you can keep track of numerical data (like temperature or dosage) and ensure it’s available for future reference.
💡Pro Tip: Accurate and detailed documentation of control variables is essential for transparency and allowing others to replicate your research.
Regularly monitor and adjust control variables
Even after you’ve identified and standardized your control variables, monitoring them regularly throughout the experiment is essential. In long-term experiments, you can use ClickUp Brain to automatically document when changes are made and highlight any inconsistencies.
External factors can sometimes cause variables to shift unintentionally. For example, lab temperatures may fluctuate slightly over time, affecting your results. Regular check-ins or recalibrations during your experiment can ensure consistency.
However, this can also be time-consuming and prone to errors when done manually. A simpler alternative is to automate repetitive tasks like reporting on the status of your variables.
Using AI to automatically record variable changes allows you to record every little change without the risk of human error. ClickUp Brain can also suggest ways to manage these deviations by analyzing past data entries and noting patterns. Its tight integration with all your project data enables it to provide tailored insights for your needs.
💡Pro Tip: Maintaining a proper relational database of all your variables and how they impact your independent variable can be extremely handy when working through any issues.
Randomization
In many experiments, randomizing certain aspects of your research can help ensure that confounding variables don’t affect your results. This is particularly useful when dealing with human subjects, where factors like age, gender, or background could unintentionally influence outcomes. Randomizing participant assignments reduces the likelihood of these variables skewing your results.
For instance, in psychology experiments, randomizing participants into control and experimental groups helps balance individual differences across both groups.
Statistical control
If your research involves complex data, consider using statistical controls to account for unmeasured confounding variables. This is particularly useful in observational studies, where it might not be possible to control every external factor manually.
Statistical techniques, such as using a regression model or covariance, allow you to mathematically control for confounding variables. This isolates the effect of your independent variable.
💡 Pro Tip: For researchers working with large datasets, ClickUp Table View and ClickUp Formulas can help automate these calculations.
Elevate Your Research with ClickUp
Mistakes with control variables can happen to anyone, but they don’t have to derail your work. By planning ahead, staying consistent, and leveraging tools like ClickUp to document and monitor your variables, you can keep your experiments on track and your results reliable.
Ready to avoid these pitfalls and elevate your research?
Sign up for a free ClickUp account today and discover how seamless managing control variables can be. 🚀