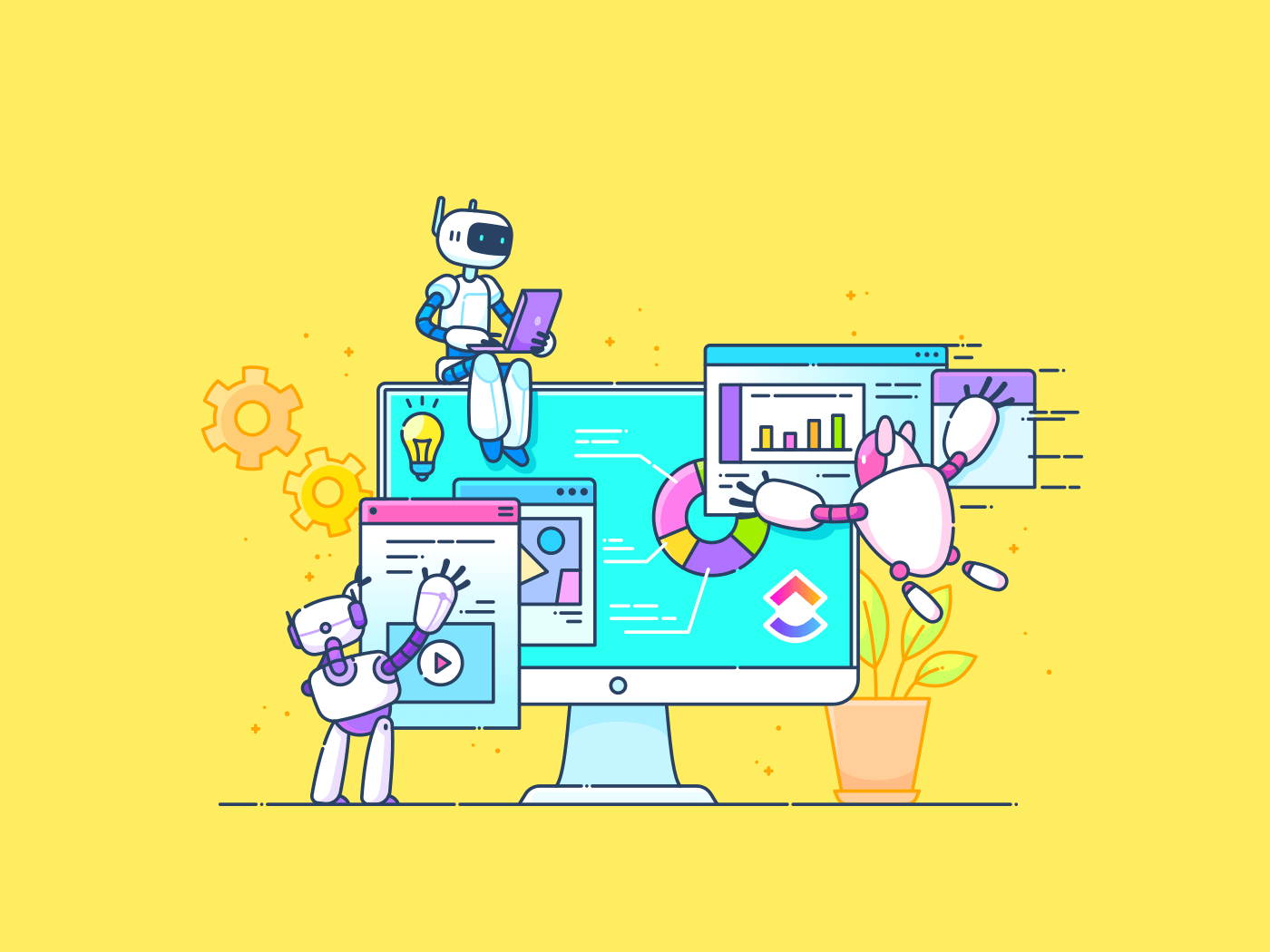
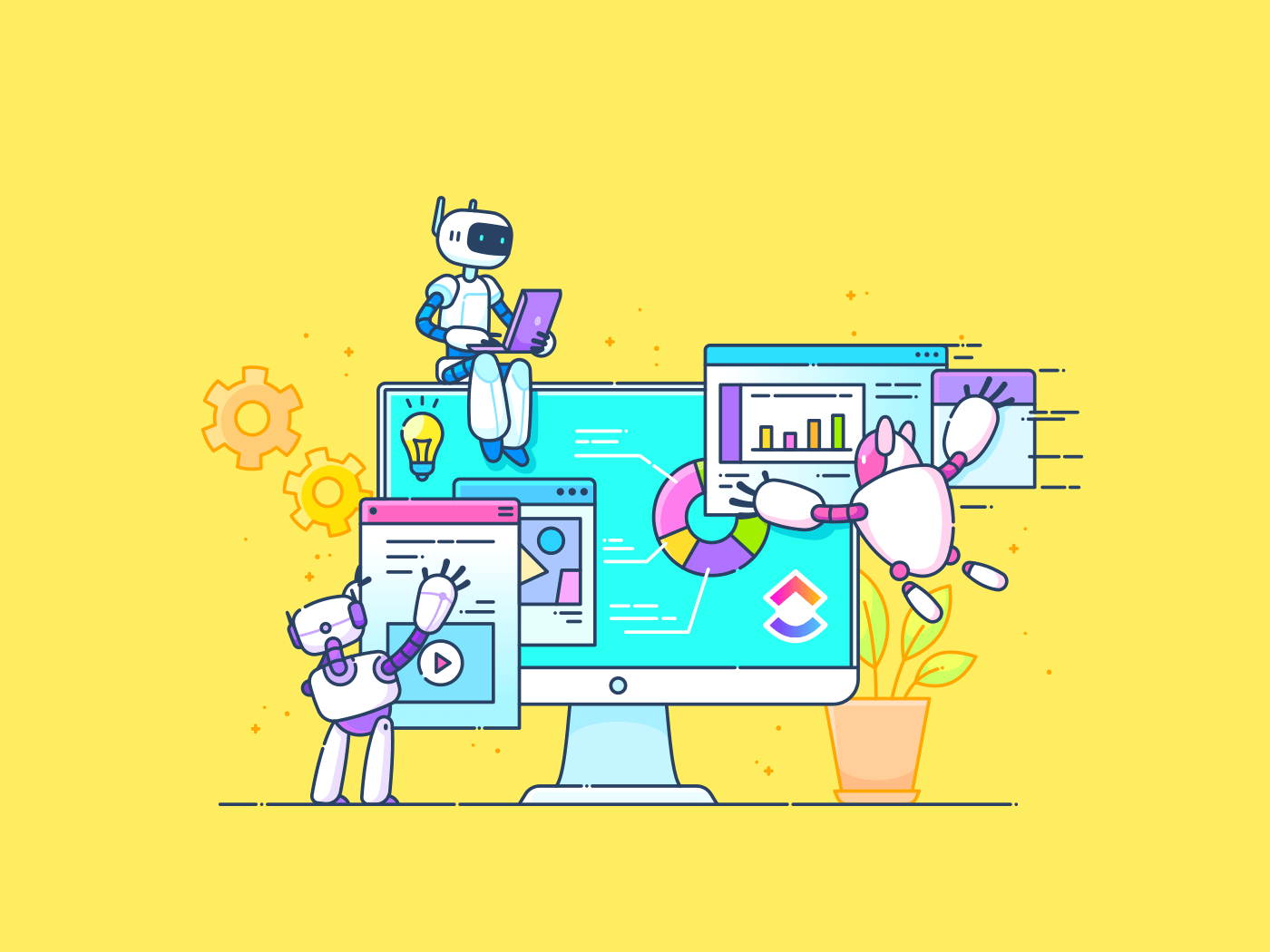
As a technology decision-maker or business leader, you know how critical it is to have accurate, timely answers.
But here’s the problem: only 20% of leaders say their organizations excel at decision-making, and most admit that a significant portion of their time is spent ineffectively, lost in the process rather than driving outcomes.
Perhaps because traditional methods—hours of research or artificial intelligence (AI) systems bound by outdated pre-trained large language models—often fall short, leaving you without the clarity you need.
That’s where the retrieval-augmented generation (RAG) truly shines.
It doesn’t just work with preloaded information but actively pulls in the most relevant, real-time data from trusted sources—internal knowledge library, external knowledge trends, industry reports, relevant documents, or customer feedback systems.
The global retrieval-augmented generation market is projected to grow at an unprecedented 44.7% CAGR by 2030, fueled by breakthroughs in natural language processing (NLP) and the rising demand for smarter artificial intelligence solutions.
Eager to see a retrieval augmented generation example? In this blog post, you’ll see how retrieval-augmented generation is already helping leaders like you personalize experiences, improve analytics, and automate critical workflows.
⏰ 60-Second Summary
- Retrieval augmented generation enhances accuracy, efficiency, and decision-making—giving you the edge in a competitive landscape
- Retrieval Augmented Generation (RAG) is an AI approach combining information retrieval and text generation
- RAG fetches relevant data from sources to generate accurate, context-aware, and informative responses.
- It helps AI produce current answers without relying on extensive training data or manual updates.
- Key retrieval augmented generation use cases include question answering, content generation, personalized recommendations, and data analysis
- Want to implement RAG? Start by defining your objectives, picking the right tools (ClickUp’s AI features work wonders here!), and measuring RAG performance
- Data quality, integration, and performance are common pain points of RAG adoption—but they’re solvable with a smart strategy
What Is Retrieval Augmented Generation (RAG)?
Retrieval-augmented generation (RAG) is a technique that combines the power of a large language model (LLM) with the ability to access and process external information.
Think of it this way: you ask a question, and instead of the AI just relying on what it’s been trained on, it pulls in real-time data sources—research papers, news articles, vector databases—and generates a highly specific, customized response.
This hybrid approach enhances AI’s capabilities by combining retrieval and generation. It ensures that the responses are relevant, fresh, and precise.
Importance of retrieval-augmented generation in enhancing AI capabilities
The real-world impact of retrieval-augmented generation is immense. Why? Because it solves one of the biggest problems with traditional AI systems: their inability to generate up-to-date answers without extensive training data or manual input.
With retrieval-augmented generation, AI can search, retrieve, and generate answers based on real-time accurate information—making it a powerful tool for anything from market research to customer service.
It makes AI far more responsive, adaptive, and, ultimately, useful because it is:
- Always current: Need answers about today’s stock prices, recent medical breakthroughs, or yesterday’s sports scores? RAG doesn’t just guess—it retrieves the exact info you need
- Deeply contextual: It’s one thing to pull in data, but RAG understands the specific context, blending facts with language so seamlessly that its responses feel like they came from a human expert
- Capable of managing complexity: Tackling problems requiring semantic search and interpretation is where RAG truly shines. It’s built for complexity, not just simple queries
How Retrieval-Augmented Generation Works
RAG’s brilliance boils down to three simple steps:
- Understanding the question: RAG doesn’t just hear your question—it figures out what you’re asking. That means grasping specific context, tone, and even subtle nuances
- Fetching the data: Using context retrieval tools, RAG dives into its connected sources, whether that’s a database, a search engine, or a library of PDFs. It’s not guessing—it’s finding
- Crafting the perfect answer: With the information retrieved, RAG’s generative AI system steps in to stitch together an accurate answer that’s articulate and tailored to your query
Key Examples of Retrieval-Augmented Generation Application
The potential of retrieval-augmented generation isn’t just theoretical—it’s already making a tangible impact across multiple industries.
Whether answering complex queries, creating personalized content, or providing insights at lightning speed, RAG proves that AI can be invaluable in real-world applications.
Here are some key ways RAG is already impacting various fields:
Question answering
Retrieval-augmented generation transforms how we get new data, especially in fields where precision and up-to-date information are crucial, such as:
Healthcare
Doctors no longer have to sift through endless studies to find the latest research on a rare disease. Retrieval-augmented generation can pull insights from the most recent medical journals and clinical studies, facilitating diagnostics and treatment decisions.
📌 Elsevier, a global medical information and data analytics company, has launched ClinicalKey AI. This tool leverages generative AI to help clinicians quickly access the latest medical research. It is designed to provide evidence-based answers to clinical questions and is optimized for natural language queries.
Legal research
Instead of digging through thick law books or outdated case law, lawyers can use retrieval-augmented generation to pull real-time legal precedents and statutes, making their research more efficient and accurate.
Customer support
Forget generic chatbot answers. Retrieval-augmented generation-enabled customer support systems can pull data from real-time sources, providing customers with specific, accurate, context-aware responses tailored to their individual needs.
📌 Grace is an AI ClickUp Assistant that helps potential and current ClickUp users solve their problems by giving them more information on ClickUp’s many productivity features and capabilities.
Content creation
Whether crafting a catchy marketing tagline or generating in-depth articles, Retrieval-augmented generation is bridging the gap between AI-generated and human content.
Here’s how it helps with content creation:
Journalism
Reporters can quickly gather relevant facts from the latest news or research. This allows them to craft stories with real-time, well-rounded information. Retrieval-augmented generation doesn’t just answer questions—it helps journalists tell timely, informed stories.
Marketing
Retrieval-augmented generation is a powerful AI tool for marketers. It helps them pull live data about trending topics, competitor activity, and consumer sentiment to craft high-performing ad campaigns or social media posts.
Education
Teachers and students alike can benefit from RAG’s ability to generate essays, reports, or even quizzes, drawing from the latest educational resources, textbooks, and online materials to ensure that content is both current and relevant.
💡Pro Tip: Train your RAG model on various creative sources, such as poems, scripts, song lyrics, or even historical documents. This diverse data pool will inspire the model to generate unique ideas.
Personalized recommendations
From shopping to entertainment, personalized recommendations powered by RAG are changing how we discover products, movies, music, and more. Here’s how:
eCommerce
Gone are the days of generic product suggestions. RAG pulls live inventory data and considers your preferences, search history, and the latest trends to offer spot-on tailored shopping recommendations.
Entertainment
RAG transforms the entertainment experience by suggesting movies, TV shows, or books based on previous preferences and real-time trends, social media buzz, and current releases.
📌 Companies like Netflix, Spotify, and Goodreads employ sophisticated recommendation systems that effectively suggest content by factoring in user history and preferences, current trends, and social media influences.
Learning platforms
Education apps are getting smarter, too, with RAG-powered systems providing personalized learning paths, curated reading lists, and content suggestions based on students’ progress and learning preferences.
Data analysis
Data is everywhere, but turning it into actionable insights can take time. With retrieval-augmented generation, data analysis is faster and more precise than ever.
Here’s how RAG helps:
Business intelligence
Retrieval-augmented generation makes AI-powered sales processes even better. It can sift through mountains of data—sales figures, market trends, customer feedback—and distill it into actionable insights, helping companies make real-time, data-driven decisions.
📌 Salesforce Einstein provides AI-powered insights by analyzing sales data and market trends, enabling businesses to make informed decisions based on predictive analytics.
Scientific research
Researchers no longer have to manually comb through thousands of academic papers to find relevant studies. RAG can analyze large datasets and extract key insights, allowing scientists to focus on groundbreaking discoveries.
Finance
In finance, RAG is invaluable for pulling live market data and news, enabling investors to make swift, informed decisions based on the latest economic trends.
📌 Bloomberg’s AI-powered earnings call summaries provide users with concise summaries and analyses of corporate performance during earnings calls. This feature is now available to all Bloomberg Terminal users, particularly focusing on companies in the Russell 1000 and the top 1000 companies in Europe. The tool aims to save analysts time by highlighting key points and providing deeper insights into financial data, helping them differentiate their research approaches.
The AI tool leverages generative AI technology, combined with insights from Bloomberg Intelligence analysts, to better understand financial language nuances. It includes critical information such as company guidance, capital allocation, labor plans, and macroeconomic factors. The integration of hyperlinks allows users to access original transcripts and related data seamlessly, enhancing transparency and the user experience.
Implementing Retrieval-Augmented Generation
Without a clear plan and the right platform, retrieval-augmented generation can become overwhelming and not deliver the expected benefits.
But how do you ensure you’re setting it up in a way that helps your team be more efficient and informed?
How can you use automation, AI, and real-time insights to drive better decision-making? And how do you make sure retrieval-augmented generation is integrated into your automated workflows without overwhelming your team?
That’s where ClickUp fits in—an all-in-one productivity platform designed to simplify task management, automate processes, and bring data-driven decision-making into your day-to-day operations.
ClickUp is built to handle conditional-logic-driven complex workflows while remaining flexible and customizable, making it an ideal choice for implementing RAG.
Here’s how you can use ClickUp to make retrieval-augmented generation work for your team:
1. Define your objectives
Determine why you need retrieval-augmented generation and the problems it will solve. Clarity in purpose ensures better outcomes, whether improving customer service through RAG chatbots, automating paragraph generation, or enhancing data analysis.
2. Identify data sources
Choose reliable and diverse sources for RAG to retrieve relevant information. Depending on your needs, these can include a vector database, APIs, or even live data streams. For example, you can train your AI customer support chatbot on your company’s existing knowledge base and help center data.
3. Select the right tools
What if there was an AI tool that could help you make smarter decisions, predict task outcomes, and suggest actions based on past data?
That’s exactly what ClickUp Brain does.
This AI feature makes your retrieval-augmented generation system smarter and more intuitive by using machine learning and advanced large language models to analyze previous projects, tasks, and even external data. This helps it generate actionable insights in real time.
Data-driven task management
Use ClickUp Brain to analyze data from previous projects, tasks, and workflows. Then, ask it to help you predict outcomes for your ongoing projects based on past patterns or what to prioritize based on urgency and importance.
Automating smart actions
Instead of manually deciding what to do with tasks based on their RAG status, use AI to build natural-language automations that can take action for you. For example, if a task is marked as ‘High Priority,’ it can be reassigned to a more qualified person.
You can do this by pairing ClickUp Automations with ClickUp Brain.
Continuous learning
As your team continues to work and complete tasks, ClickUp Brain learns and adapts, improving its recommendations. This means your retrieval-augmented generation system gets more accurate and refined over time, making it even more valuable for long-term use.
While these features can add much value, can ClickUp Brain predict task outcomes and trends?
Yes, by analyzing patterns from completed tasks and historical data, ClickUp Brain predicts delays, risks, and potential bottlenecks.
It can even predict which tasks will require more time based on data from similar past projects. This predictive capability is crucial for effective retrieval-augmented generation implementation and strategic project management because it helps you make adjustments before small problems escalate into bigger ones.
4. Integrate RAG into workflows
Ensure seamless alignment between RAG processes and existing operations. Finetune the retrieval model for relevance and accuracy based on dynamic data and your industry requirements.
5. Test and refine
Run pilot tests to evaluate the effectiveness of your retrieval-augmented generation system. Continuously improve performance by incorporating feedback and addressing gaps in retrieval or generation.
6. Monitor and scale
Monitor your retrieval-augmented generation system regularly to ensure it remains accurate and efficient. Once it has proven effective, scale it to other areas of your organization for broader impact.
So, how do you keep track of your team’s tasks and projects in a way that reflects each team member’s true status? How can you ensure you know exactly which tasks need attention and which are on track without constantly checking in on each one?
Use the ClickUp RAG Reporting Template
The ClickUp RAG Reporting Template, a simple yet powerful tool, can handle this.
This template categorizes tasks based on their status—Red (urgent issues), Amber (in-progress tasks), and Green (on track). This color-coded system is intuitive and makes it easy to see at a glance where attention is needed.
But how does this template integrate into your retrieval-augmented generation system?
Here’s a primer:
- Real-time task updates: The template auto-updates as your team progresses through tasks. This means that once tasks are marked ‘Red’ due to delays or issues, the system immediately flags them, alerting your team to prioritize them
- Customizable for your needs: It is fully customizable. You can adjust how the ‘Red,’ ‘Amber,’ and ‘Green’ statuses are triggered, allowing the template to match how your team works
- Clear communication across teams: When tasks are color-coded, it’s easy for everyone on the team to understand what needs to be prioritized quickly
Can the RAG Reporting Template scale across multiple projects and teams?
Yes! Whether managing a small team or working across multiple departments, the template can scale to meet your needs.
You can create separate retrieval-augmented generation templates for different projects, clients, or departments and then consolidate them in one dashboard for an overview of everything happening simultaneously.
This way, you can manage and track complex workflows without losing sight of important details.
💡 Pro Tip: While RAG can be valuable, human judgment remains crucial. Maintaining a review process ensures the generated content aligns with ethical guidelines and avoids perpetuating biases.
Challenges and solutions during RAG implementation
RAG has amazing potential, but putting it into action isn’t always smooth sailing. Here are some common challenges and how to tackle them:
Messy or outdated data
Bad data equals bad answers. Retrieval-augmented generation relies on clean, current information to work well. If the data is outdated or irrelevant, the quality of generated content will suffer, leading to less accurate or useful outputs.
Solution: Regularly update sources and filter out unreliable content. Prioritize high-quality, trusted sources over volume to ensure that the AI can retrieve and use only the most relevant information. This helps the system generate more accurate and timely answers.
Slow response times
Real-time data retrieval can lag, especially when large datasets are involved or when accessing external sources takes time, frustrating users with delays in getting responses.
Solution: Use caching strategies for frequently accessed data to reduce retrieval times. Additionally, optimizing semantic search algorithms and leveraging indexing techniques can help speed up the retrieval process and improve response times for users.
Mismatch between retrieved and generated content
Sometimes, the pieces don’t fit, leading to clunky answers that fail to address the user’s query effectively.
Solution: Fine-tuning the AI model through supervised learning can help ensure that the generated content is better aligned with the retrieved data. Adding layers of context or employing post-processing techniques can also smooth out mismatches, leading to more cohesive and relevant responses.
Data privacy worries
With the increasing use of sensitive data in RAG systems, there are concerns about data breaches or mishandling, especially when personal or confidential information is involved.
Solution: Implement strong data protection measures such as encryption, anonymization of sensitive data, and regular audits to ensure compliance with privacy laws like GDPR. By safeguarding user data, organizations can minimize privacy risks and build trust with their users.
High costs and scalability
As RAG systems scale, the infrastructure costs can quickly spiral due to the need for powerful hardware, increased data storage, and higher processing power, making it difficult to sustain large-scale implementations.
Solution: Leverage cloud-based platforms that allow for elastic scaling, which helps manage costs more effectively. Additionally, simplifying queries and optimizing retrieval methods can reduce computational requirements, making the system more cost-efficient as it grows.
Benefits of Using RAG
Despite its challenges, the advantages of RAG make it a compelling choice for various industries.
Let’s explore how RAG delivers value:
- Always up-to-date: RAG brings you fresh, real-world insights instead of relying on static, pre-trained data
- Provides more accurate answers: By combining retrieval with the generation, RAG ensures responses are both precise and contextually relevant
- Helps make smarter decisions: RAG delivers detailed insights, helping teams make better calls faster
- Provides personalization at scale: RAG tailors results to each user, creating unique and relevant experiences
- Saves time and effort: By automating research and generating the content, RAG lightens the load
- Works everywhere: From eCommerce to disaster relief, RAG is versatile enough to make a difference in any industry
RAG + ClickUp: Your Blueprint for Smarter Workflows
The power of retrieval-augmented generation lies in the technology and its application to real-world challenges. From enhancing customer service to creating highly relevant content, empowering semnatic search, and even streamlining research, RAG is a tool that thrives on the right implementation.
With ClickUp Brain, you can use the true potential of retrieval-augmented generation by automating decisions, identifying bottlenecks, and leveraging actionable insights from real-time data.
Pair this with ClickUp’s RAG Reporting Template, and you’ve got a visual, dynamic, connected AI system to track priorities, address issues before they escalate, and keep your projects in the ‘Green.’
Sign up to ClickUp today!