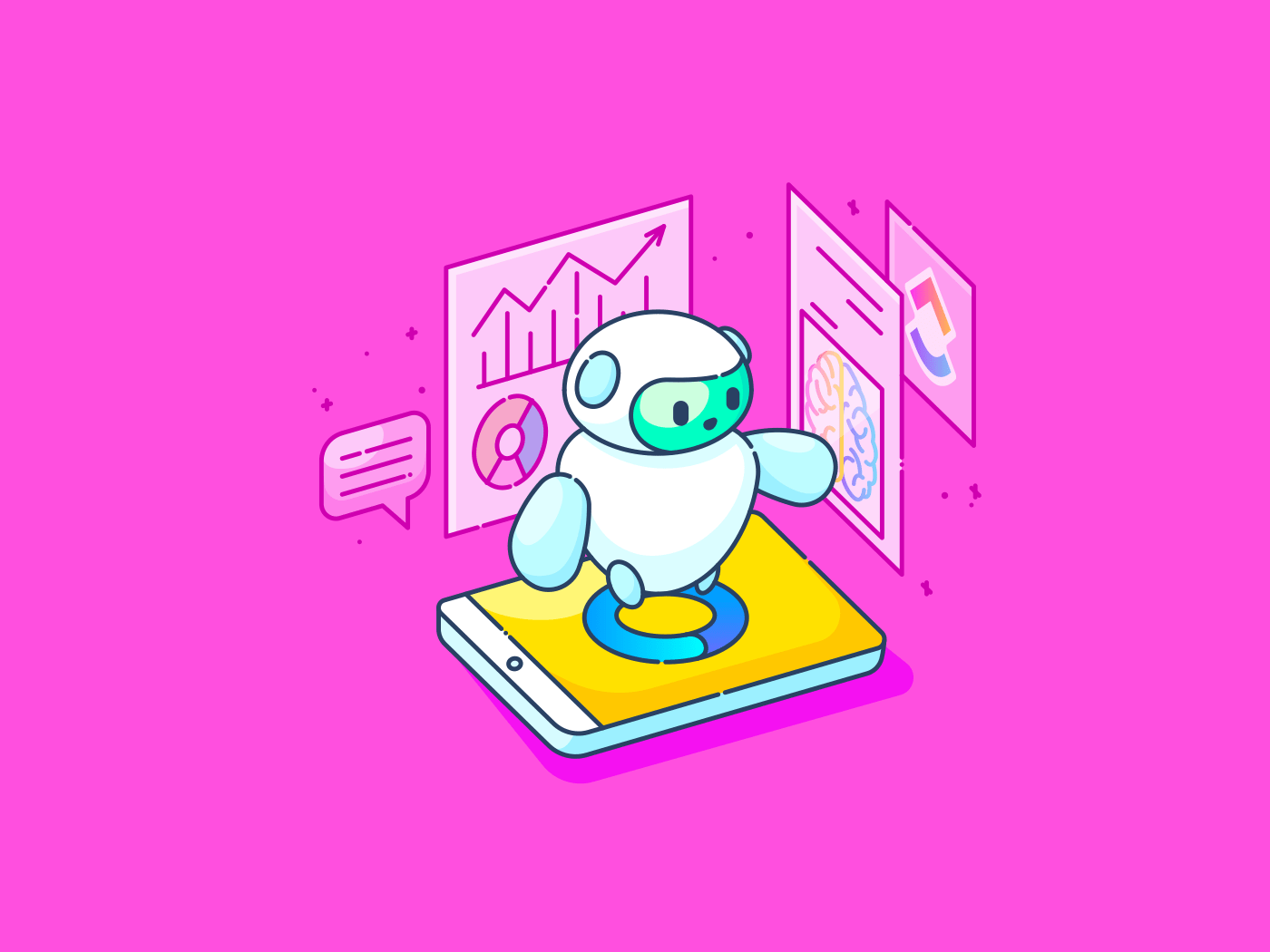
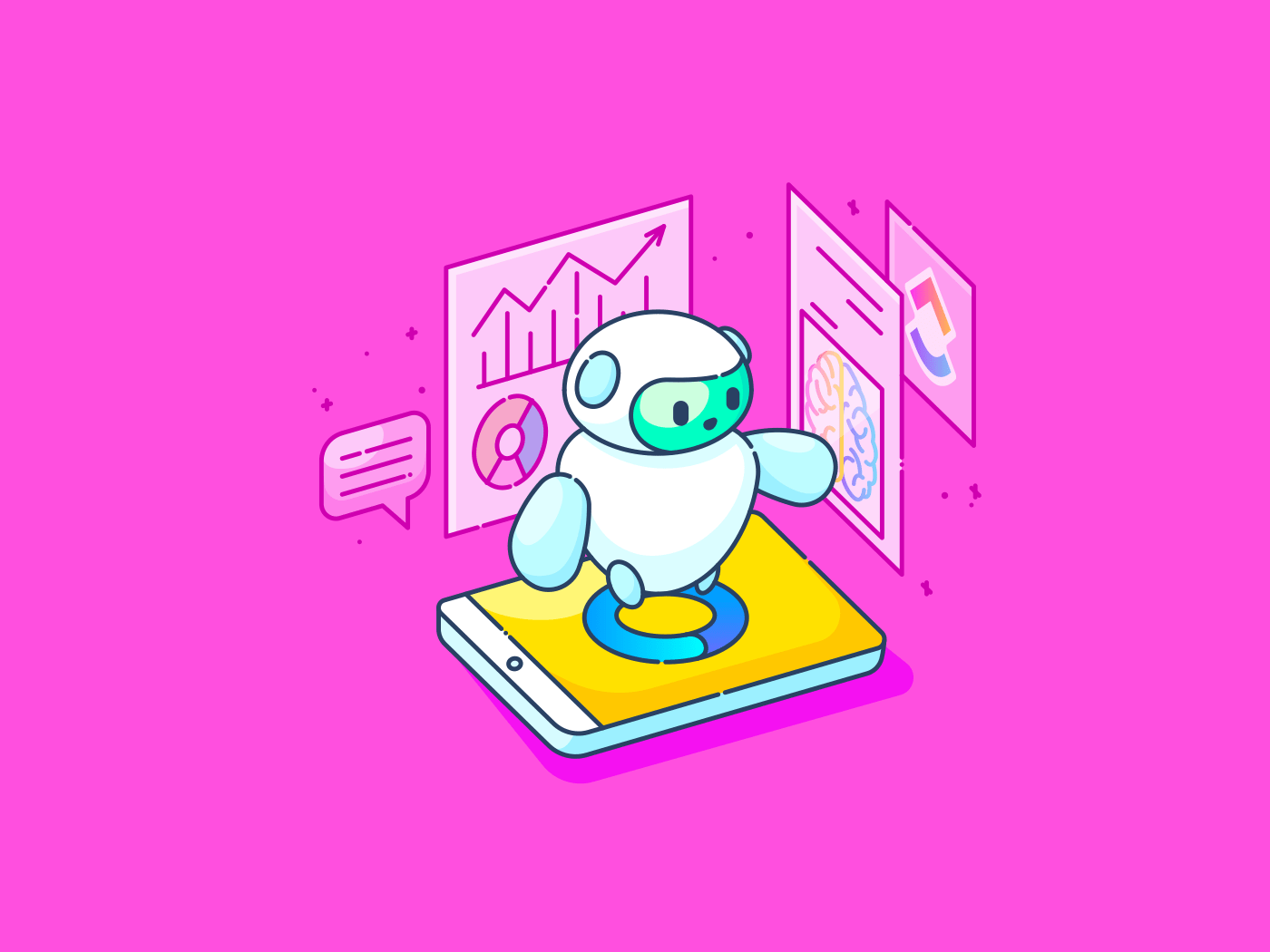
Most of us have had some experience ‘talking’ with the latest AI tools on the block. If you’ve spent enough time with AI, you already know it’s like that brilliant but forgetful friend who has great ideas but sometimes forgets what you two talked about. Or that always-on-the-phone colleague who shares dubious news reports from random chat threads, spreading misinformation.
That’s just the tip of the iceberg when we talk about challenges in artificial intelligence.
Researchers from Oregon State University and Adobe are developing a new training technique to reduce social bias in AI systems. If this technique proves reliable, it could make AI fairer for everyone.
But let’s not get ahead of ourselves. This is just one solution among many needed to tackle the numerous AI challenges we face today. From technical hitches to ethical quandaries, the road to reliable AI is paved with complex issues.
Let’s unpack these AI challenges together and see what it takes to overcome them.
- 10 AI Challenges and Solutions
- 1. Algorithmic bias
- 2. Lack of AI transparency causing distrust
- 3. Scaling AI is tougher than it looks
- 4. Deepfake and generative AI fraud
- 5. Interoperability and human-AI interaction challenges
- 6. AI ethics is about more than just good intentions
- 7. Mixing up AI data sets could spell disaster
- 8. Risks & concerns with automated decision-making
- 9. Lack of clear AI rules and regulations
- 10. Misinformation from AI
- Tools for Dealing with AI Challenges
- Meeting AI Challenges with the Right Tools
10 AI Challenges and Solutions
As AI technology advances, it confronts a range of issues. This list explores ten pressing AI challenges and outlines practical solutions for responsible and efficient AI deployment.
1. Algorithmic bias
Algorithmic bias refers to the tendency of AI systems to exhibit biased outputs, often due to the nature of their training data or design. These biases can manifest in numerous forms, often perpetuating and amplifying existing societal biases.
An example of this was observed in an academic study involving the generative AI art generation application Midjourney. The study revealed that when generating images of people in various professions, the AI disproportionately depicted older professionals with specialized job titles (e.g., analyst) as male, highlighting a gender bias in its output.
Solutions
- Diverse and representative data: Use training datasets that truly reflect the diversity of all groups to avoid biases related to gender, ethnicity, or age
- Bias detection and monitoring: Regularly check your AI systems for biases. This should be a combination of automated monitoring and your own manual reviews to ensure nothing slips through
- Algorithmic adjustments: Take an active role in adjusting AI algorithms to fight bias. This could mean rebalancing the data weights or adding fairness constraints to your models
- Ethical AI guidelines: Help shape ethical AI practices by adopting and implementing guidelines that tackle fairness and bias, ensuring these principles are woven into every stage of your AI project
2. Lack of AI transparency causing distrust
Transparency in AI means being open about how AI systems operate, including their design, the data they use, and their decision-making processes. Explainability goes a step further by ensuring that anyone, regardless of their tech skills, can understand what decisions AI is making and why. These concepts help tackle fears about AI, such as biases, privacy issues, or even risks like autonomous military uses.
Understanding AI decisions is crucial in areas like finance, healthcare, and automotive, where they have significant impacts. This is tough because AI often acts as a ‘black box’—even its creators can struggle to pinpoint how it makes its decisions.
Solutions
- Develop clear documentation: Provide comprehensive details about AI models, their development process, data inputs, and decision-making processes. This fosters a better understanding and sets a foundation for trust
- Implementing AI models that are explainable: Utilize models that provide more transparency, such as decision trees or rule-based systems, so users see exactly how inputs are turned into outputs
- Use interpretability tools: Apply tools like LIME (Local Interpretable Model-agnostic Explanations) or SHAP (SHapley Additive exPlanations) to break down the contributions of various features in the model’s decision-making process
3. Scaling AI is tougher than it looks
Scaling AI technology is pivotal for organizations aiming to capitalize on its potential across various business units. However, achieving this scalability of AI infrastructure is fraught with complexities.
According to Accenture, 75% of business leaders feel that they will be out of business in five years if they cannot figure out how to scale AI.
Despite the potential for a high return on investment, many companies find it difficult to move beyond pilot projects into full-scale deployment.
Zillow’s home-flipping fiasco is a stark reminder of AI scalability challenges. Their AI, aimed at predicting house prices for profit, had error rates up to 6.9%, leading to severe financial losses and a $304 million inventory write-down.
The scalability challenge is most apparent outside of tech giants like Google and Amazon, which possess the resources to leverage AI effectively. For most others, especially non-tech companies just beginning to explore AI, the barriers include a lack of infrastructure, computing power, expertise, and strategic implementation.
Solutions
- Enhanced infrastructure: Develop a robust digital infrastructure that can handle large-scale AI deployments. For instance, cloud services and localized data centers reduce latency and improve performance
- Cross-disciplinary teams: Foster a collaborative environment where tech and business units work together to integrate AI solutions seamlessly into existing business models
- Automated AI development tools: Utilize platforms like TurinTech’s evoML to automate the development of machine learning codes, enabling faster model creation and deployment
- Continuous learning and adaptation: Implement mechanisms for continuous learning and updating of AI models to adapt to real-world data and changing market conditions, ensuring long-term relevance and efficiency
- Invest in talent development: Build internal AI expertise through training and hiring practices that focus on emerging AI technologies, reducing over-reliance on external AI talent
Also read: Essential AI stats to know today
4. Deepfake and generative AI fraud
Generative AI and deepfake technologies are transforming the fraud landscape, especially in the financial services sector. They make it easier and cheaper to create convincing fakes.
For example, in January 2024, a deepfake impersonating a CFO instructed an employee to transfer $25 million, showcasing the severe implications of such technologies.
This rising trend highlights the challenges banks face as they struggle to adapt their data management and fraud detection systems to counter increasingly sophisticated scams that not only deceive individuals but also machine-based security systems.
The potential for such fraud is expanding rapidly, with projections suggesting that generative AI could push related financial losses in the U.S. to as high as $40 billion by 2027, a significant leap from $12.3 billion in 2023.
Solutions
- Advanced detection technologies: Banks must invest in newer technologies that can detect deepfake and generative AI anomalies more effectively
- Human oversight: Integrating skilled human analysis with AI responses enhances detection rates and helps verify and understand AI-driven fraud identification
- Collaborative fraud prevention efforts: Establishing partnerships within and across industries can help develop more robust mechanisms to identify and prevent fraud
5. Interoperability and human-AI interaction challenges
When different organizations or countries use AI together, they must ensure that AI behaves ethically according to everyone’s rules. This is called ethical interoperability, and it’s especially important in areas like defense and security.
Right now, governments and organizations have their own set of rules and ethos. For instance, check out Microsoft’s Guidelines for Human-AI Interaction:
However, there’s a lack of standardization in this ethos and rules across the globe.
Right now, AI systems come with their own set of ethical rules, which might be okay in one place but problematic in another. When these systems interact with humans, if they don’t behave as expected, it can lead to misunderstandings or mistrust.
Solutions
- Set universal ethical standards: Agree on basic ethical rules that all AI systems must follow, no matter where they come from. Focus on fairness, accountability, and transparency
- Use a strong certification system: Before any AI system is used, it should pass a tough test to confirm it meets these ethical standards. This could include checks by the creators and also by independent groups
- Ensure everyone is in the loop: Always be clear about how the AI makes decisions and uses data. This transparency helps build trust and makes it easier to integrate different AI systems
- Keep an eye on things: Regularly check the AI systems to make sure they continue to meet ethical standards. Update them as needed to keep up with new rules or technologies
6. AI ethics is about more than just good intentions
Artificial Intelligence (AI) is zooming into almost every part of our lives—from self-driving cars to virtual assistants, and it’s brilliant! But here’s the catch: how we use AI can sometimes stir up serious ethical headaches. There are thorny ethical issues around privacy, bias, job displacement, and more.
With AI being able to do tasks humans used to do, there’s a whole debate about whether it should even be doing some of them.
For example, should AI write movie scripts? Sounds cool, but it sparked a massive stir in the entertainment world with strikes across the USA and Europe. And it’s not just about what jobs AI can take over; it’s also about how it uses our data, makes decisions, and sometimes even gets things wrong. This has everyone from tech builders to legal eagles hustling to figure out how to handle AI responsibly.
Solutions
- Clarify the rules: Develop crystal-clear guidelines on how AI should be used. This means setting boundaries to prevent misuse and understanding the legal implications of AI’s actions
- Respect privacy: Huge amounts of data, including personal information, are used to train AI. We need to be super careful about how this data is collected, used, and protected. This is about making sure AI respects our privacy
- Fight bias: AI is only as good as the data it learns from, and sometimes this data has biases. We must scrub these biases from AI systems to make sure they’re fair and don’t discriminate
- Protect intellectual property: AI can churn out work based on what it’s learned from others’ creative works. This can tread on copyrights and rob creators of their due unless we watch out
- Ethics vs. speed: In the mad dash to get the latest AI technologies to market, ethics can get sidelined. We’ve got to balance the need for speed with doing things right
7. Mixing up AI data sets could spell disaster
When developing AI machine learning models, it can be challenging to distinguish correctly between training, validation, and testing datasets. The AI model training dataset teaches the model, the validation dataset tunes it, and the testing dataset evaluates its performance.
Mismanagement in splitting these datasets can lead to models that either fail to perform adequately due to underfitting or perform too well on training data but poorly on new, unseen data due to overfitting.
This misstep can severely hamper the model’s ability to function effectively in real-world AI applications, where adaptability and accuracy on standardized data are key.
Solutions
- Structured data splitting: Adopt a systematic approach to divide data into training, validation, and testing sets
- Cross-validation techniques: Utilize cross-validation methods, especially in scenarios with limited data. Techniques like K-fold cross-validation help maximize the training usage and provide a more robust estimate of the model’s performance on unseen data
- Data randomization: Ensure that the data split is randomized to prevent any AI bias from being introduced by the order of the data. This helps in creating training and validation sets that are representative of the overall dataset
8. Risks & concerns with automated decision-making
When AI makes decisions, things can get tricky, especially in critical areas like healthcare and banking. One big problem is that we can’t always see how AI systems come up with their decisions.
This can lead to unfair decisions that nobody can explain. Plus, these systems are targets for hackers who, if they get in, could steal a lot of important data.
Solutions
- Develop robust security protocols: Ensure AI systems are locked down tight against hackers. Keep updating security to close any new loopholes that pop up
- Enhance transparency: Use tech that helps AI explain its choices in simple terms. If everyone understands how decisions are made, they’ll trust AI more
- Protect private information: Secure all the personal data that AI handles. Follow laws like the GDPR to make sure no one’s privacy is compromised
- Foster multi-disciplinary collaboration: Get experts from all fields—tech, law, ethics—to work together. They can help make sure AI decisions are fair and safe
Also read: The most popular AI tools for students
9. Lack of clear AI rules and regulations
Right now, there isn’t a single global watchdog for AI; regulation varies by country and even by sector. For example, there’s no central body specifically for AI in the US.
What we see today is a patchwork of AI governance and regulations enforced by different agencies based on their domain—like consumer protection or data privacy.
This decentralized approach can lead to inconsistencies and confusion; different standards may apply depending on where and how AI is deployed. This makes it challenging for AI developers and users to ensure they’re fully compliant across all jurisdictions.
Solutions
- Establish a dedicated AI regulatory body: Countries could benefit from setting up a specific agency focused on AI. This body could oversee all AI-related issues, keeping up with the fast pace of AI development and ensuring compliance with safety and ethical standards
- International cooperation: AI doesn’t stop at borders. Countries need to work together to create international standards and agreements on AI use, similar to how global treaties work for environmental protection
- Clear and adaptive legislation: Laws need to be clear (so that companies know how to comply) but also flexible enough to adapt to new AI advancements. Regular updates and reviews of AI laws could help keep them relevant
- Public and stakeholder involvement: Regulations should be developed with input from a wide range of stakeholders, including tech companies, ethicists, and the general public. This can help ensure that diverse viewpoints are considered and that the public trusts AI systems more
Also read: AI tools for lawyers
10. Misinformation from AI
Imagine having technology that can think like a human. That’s the promise of Artificial General Intelligence (AGI), but it comes with big risks. Misinformation is one of the main issues here.
With AGI, one can easily create fake news or convincing false information, making it harder for everyone to figure out what’s true and what’s not.
Plus, if AGI makes decisions based on this false info, it can lead to disastrous outcomes, affecting everything from politics to personal lives.
Solutions
- Set up strong checks: Always double-check facts before letting AGI spread information. Use reliable sources and confirm the details before anything goes public
- Teach AGI about ethics: Just like we teach kids right from wrong, we need to teach AGI about ethical behavior. This includes understanding the impact of spreading false information and making decisions that are fair and just
- Keep humans in the loop: No matter how smart AGI gets, keep humans involved in the decision-making process. This helps catch mistakes and ensures that AGI’s actions reflect our values and ethics
- Create clear rules: Set up strict guidelines for what AGI can and can’t do, especially when it comes to creating and spreading information. Make sure these rules are followed to the letter
Also read: The complete AI glossary
Tools for Dealing with AI Challenges
When you’re knee-deep in AI, picking the right tools isn’t just a nice-to-have; it’s a must to ensure your AI journey doesn’t turn disastrous. It’s about simplifying the complex, securing your data, and getting the support you need to solve AI challenges without breaking the bank.
The key is to choose tailored AI software that enhances productivity while also safeguarding your privacy and the security of your data.
Enter ClickUp Brain, the Swiss Army knife for AI in your workplace.
ClickUp Brain: Efficiency, security, and innovation—all rolled into one
ClickUp Brain is designed to handle everything AI-related—from managing your projects and documents to enhancing team communication. With the AI capabilities of ClickUp Brain, you can tackle data-related challenges, improve project management, and boost productivity, all while keeping things simple and secure. ClickUp Brain is a comprehensive solution that:
- Integrates seamlessly into your daily work
- Ensures your data remains your own
- Saves your money and resources
ClickUp Brain integrates intelligently into your workflow to save you time and effort while also safeguarding your data. It is (like the rest of the ClickUp platform) GDPR compliant, and doesn’t use your data for training.
Here’s how it works:
- AI Knowledge Manager: Ever wished you could get instant, accurate answers from your work documents or chats? ClickUp Brain makes it possible. No more digging through files for hours. Just ask, and you shall receive—whether it’s details from a project doc or insights from past team updates
- AI Project Manager: Imagine having an AI sidekick that keeps your projects on track. From automating task updates to summarizing project progress, ClickUp Brain handles the tedious parts so you can focus on the big picture
- AI Writer for Work: This tool is a game-changer for anyone who dreads writing. Whether you’re drafting a quick email or crafting a comprehensive report, ClickUp Brain helps refine your prose, check your spelling, and adjust your tone to perfection
Meeting AI Challenges with the Right Tools
Despite the AI challenges we’ve discussed, we can agree that artificial intelligence has come a long way. It has evolved from basic automation to sophisticated systems that can learn, adapt, and predict outcomes. Many of us have now integrated AI into various aspects of our lives, from virtual assistants to advanced data collection and analysis tools.
As AI advances, we can expect even more innovations, AI hacks, and AI tools to enhance productivity, improve decision-making, and revolutionize industries. This progress opens up new possibilities, driving us toward a future where AI plays a crucial role in both personal and professional spheres.
With AI tools like ClickUp Brain, you can make the most of AI technologies while also securing yourself against AI challenges to privacy and data security. ClickUp is your go-to AI-powered task management tool for everything from software projects to marketing. Choose ClickUp to securely transform your organization into a data and AI-powered entity while you grow team productivity.
Ready to transform your workflows with AI? Sign up for ClickUp now!