The only AI that
works with your work
Make AI your own
Your agents
AI tools
Your workflows
Your data
Save 1 day per week, guaranteed.
150,000+ teams get an unfair advantage using ClickUp Brain
AI in every feature
Your knowledge.
Your workflows. Your AI.
AI SyncUps & Notetaker
Start with your idea
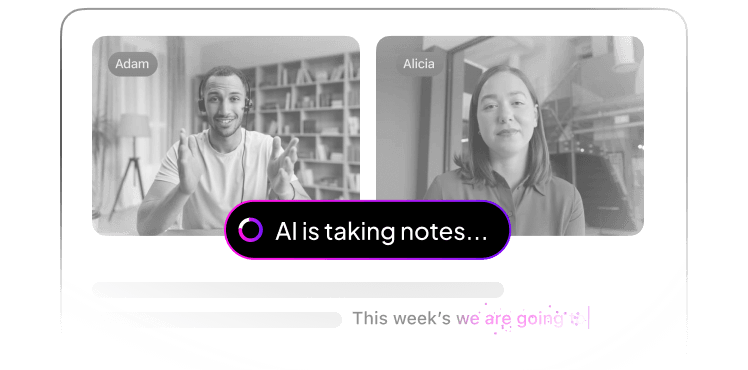
AI Tools
Brain creates the brief and tasks
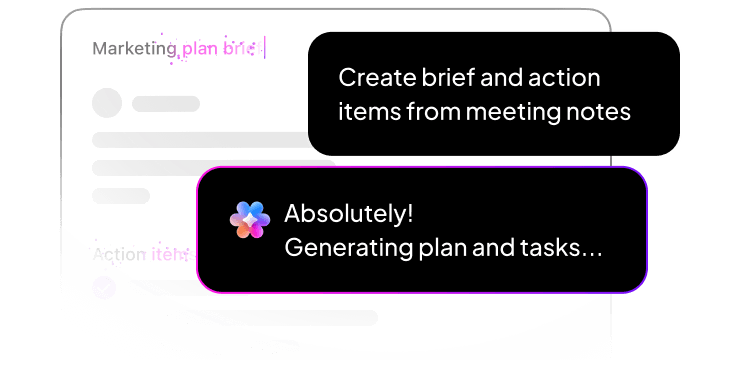
AI Image Generator
Brain Agents jump in to design
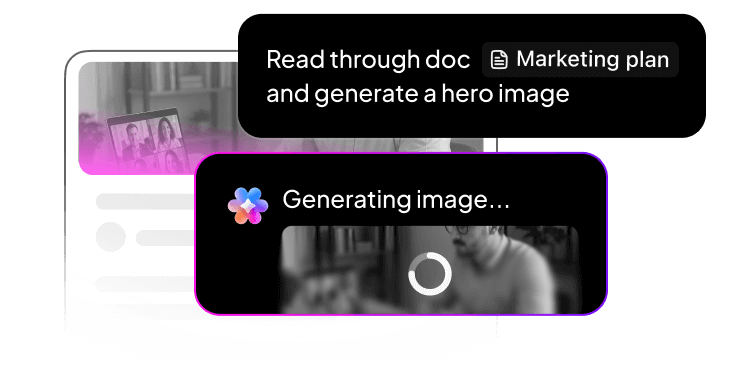
AI Stand-ups & Answers Agent
Brain loops everyone in and answer questions
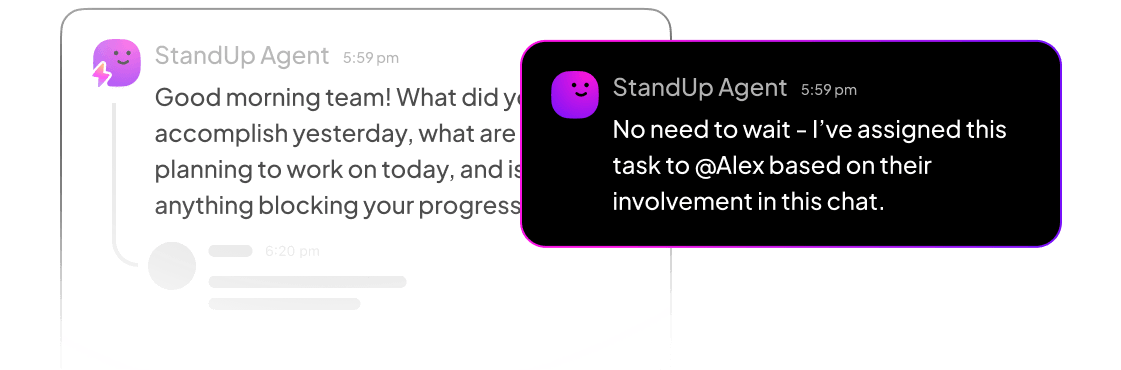
AI Autopilot
Brain prioritizes and delegates
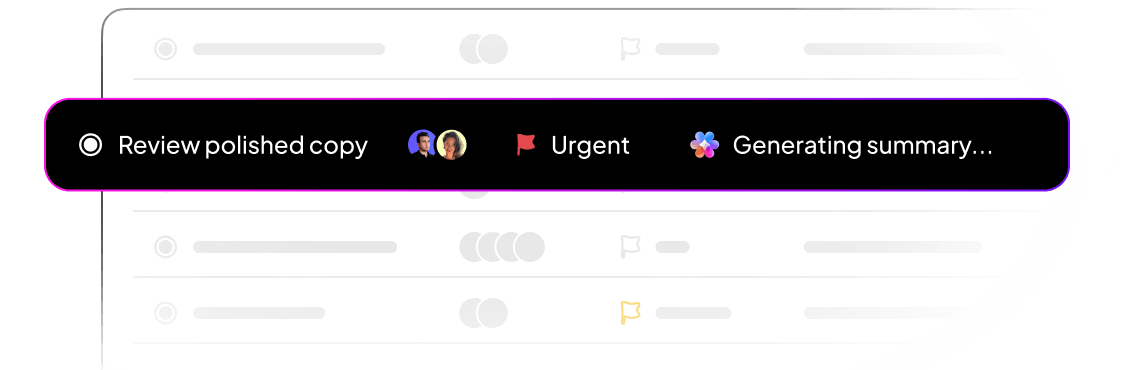
All-in-one AI, right where you work
Accessible from anywhere. Connected to your work and apps.
The complete end-to-end AI stack
Transform your business with best-in-class AI tools
Autonomous Projects
Work moves forward on its own with task assigning, progress tracking, and prioritization.
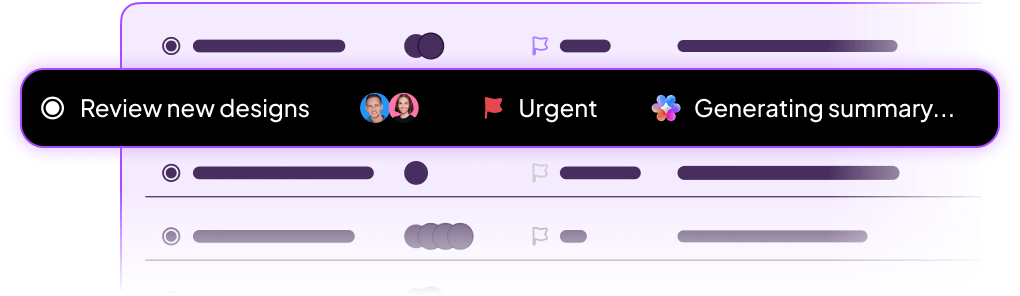
Enterprise AI Search & Ask
Get trusted answers from every corner of your Workspace, instantly and in full context.
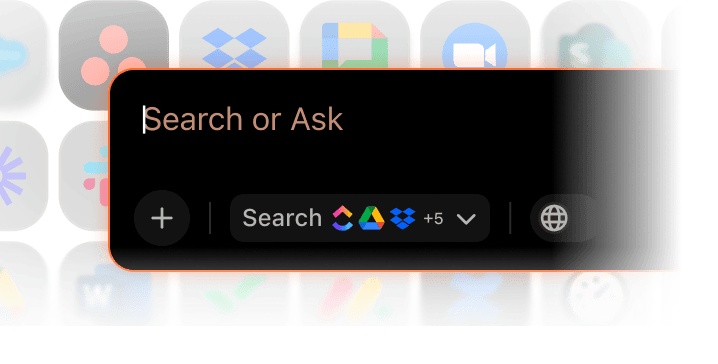
AI Agents & Assistants
Offload busywork to AI agents that turbocharge output
Autonomous Answers
Delivers instant, context‑rich answers that keep teams moving and up-to-date.
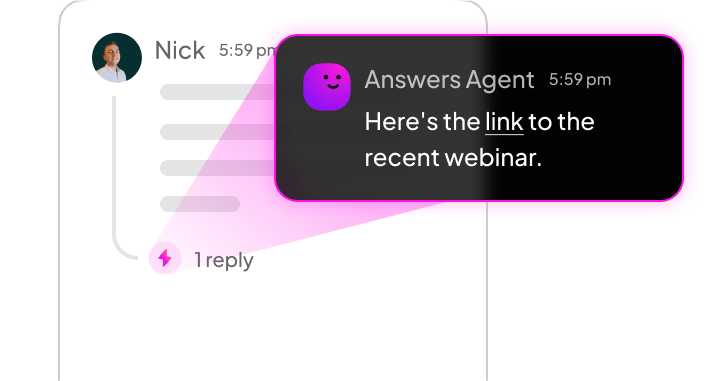
Autopilot Agents
Define workflows and triggers, then let Brain handle progress tracking and reports.
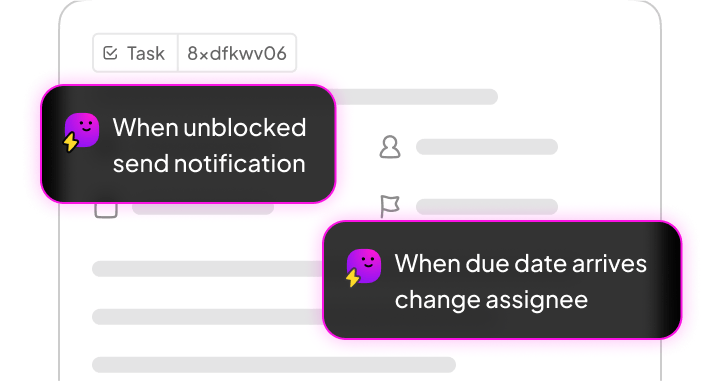
Custom Agents
Build custom AI agents and assistants for any task without writing a line of code.
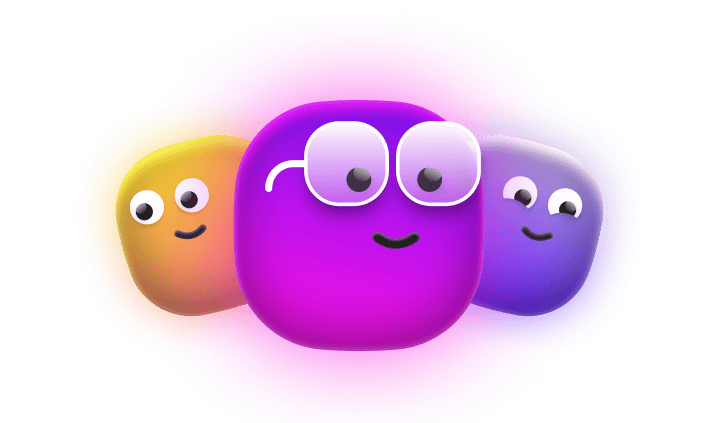
AI Project Management
Autonomous projects without the busywork or micromanagement
Auto-Tracking Progress
Everything gets tracked for you, from risks to milestones. No follow-ups, no check-ins.
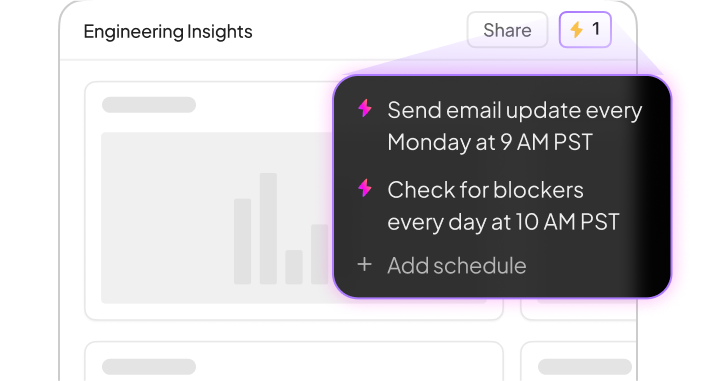
AI Stand-ups
Daily tasks and blockers are compiled into one clear, meeting-free summary.
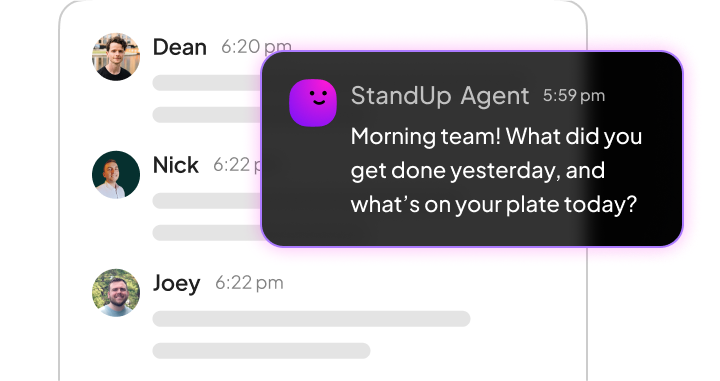
Intelligent Task Assigning
AI assigns each task to the right person based on expertise, availability, and workload.
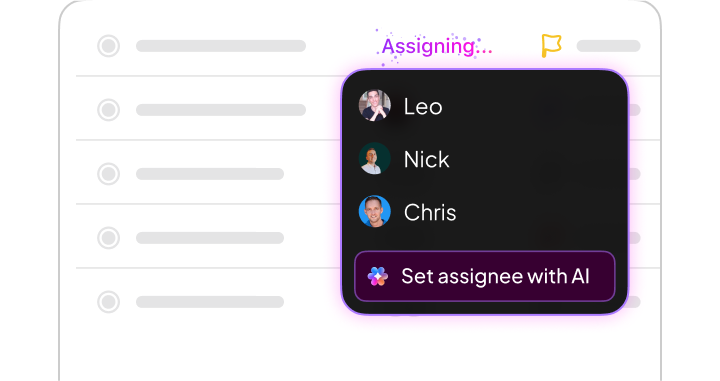
Self-Prioritizing Tasks
High-impact work stays in focus because priorities adapt automatically as things move.
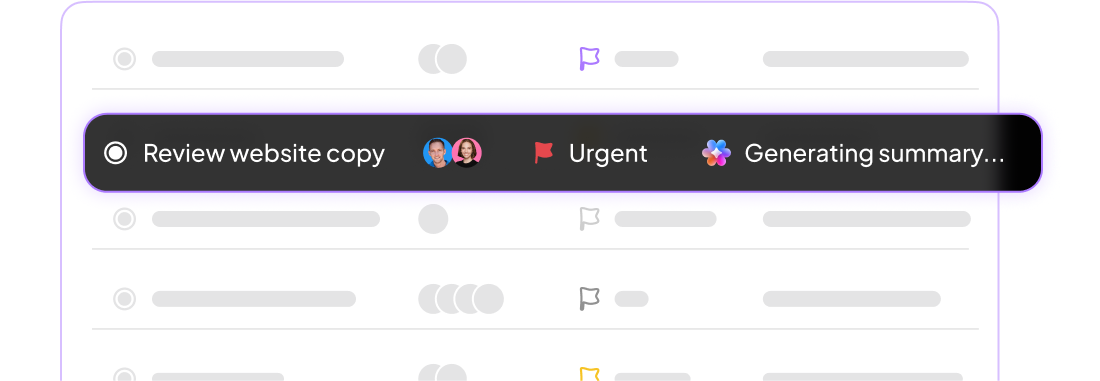
Fields That Fill Themselves
From statuses to summaries, project data updates itself so you never lift a finger.
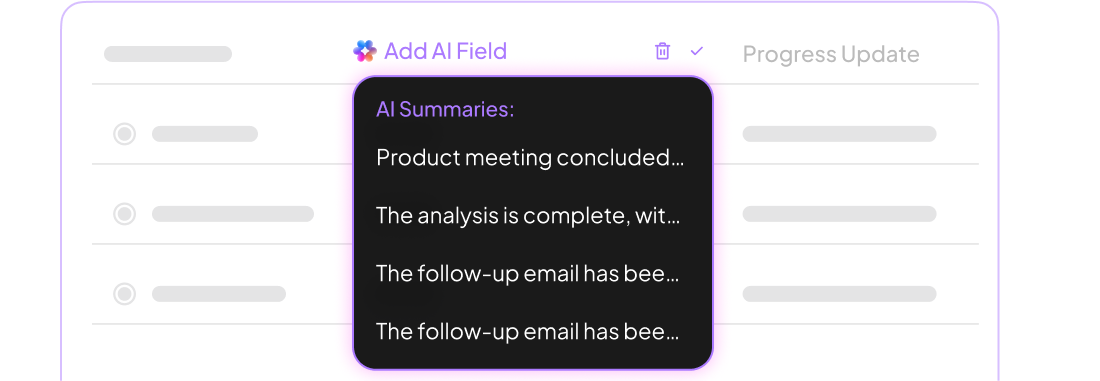
AI Meetings
Free your team from note-taking, scheduling, and status updates
Meetings That Take Notes
Everything said is auto-captured and turned into docs, tasks, and follow-ups.
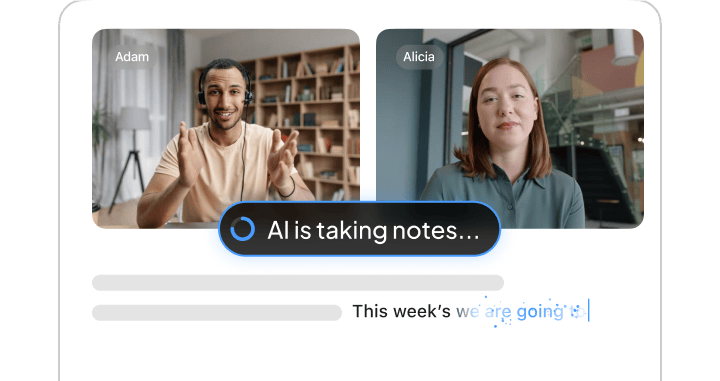
Smart Clip Recordings
AI turns your clips into shareable, searchable content, no effort required.
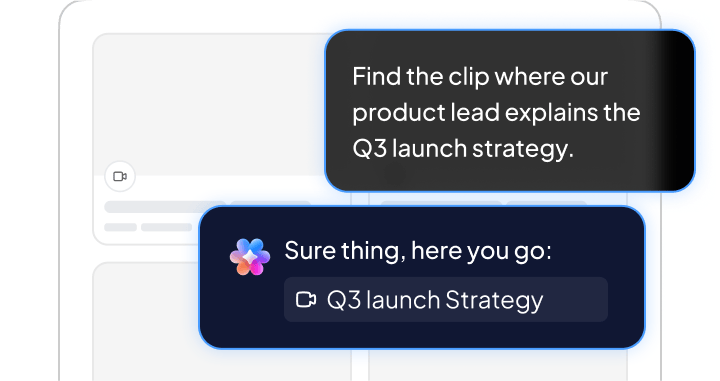
Scheduling Without the Back-and-Forth
Let AI find the best time, book meetings, and sync with your calendars.
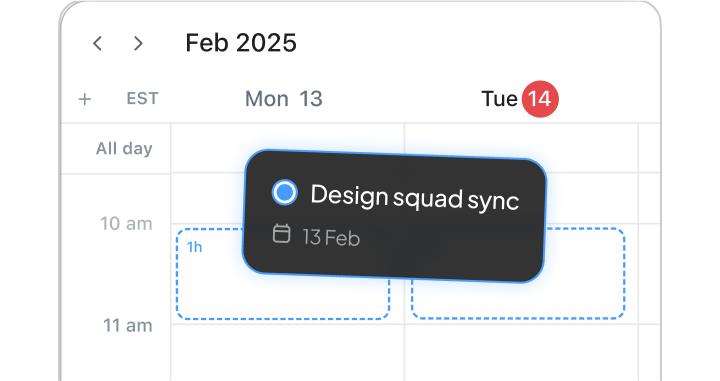
AI-Powered SyncUp Meetings
Every call becomes a searchable transcript, summary, and list of next steps—automatically.
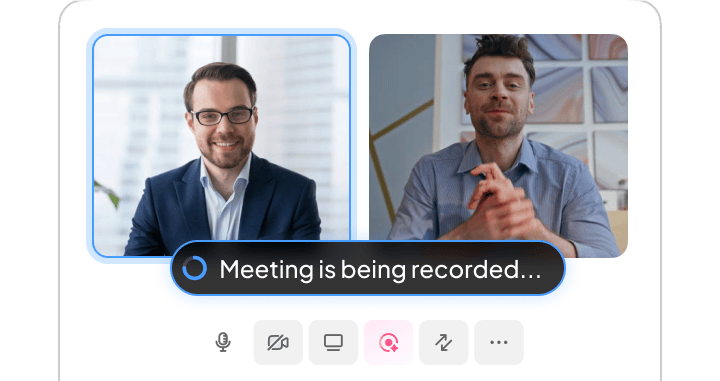
Answers Without the Search
Ask anything in a meeting and get instant AI answers from your Workspace.
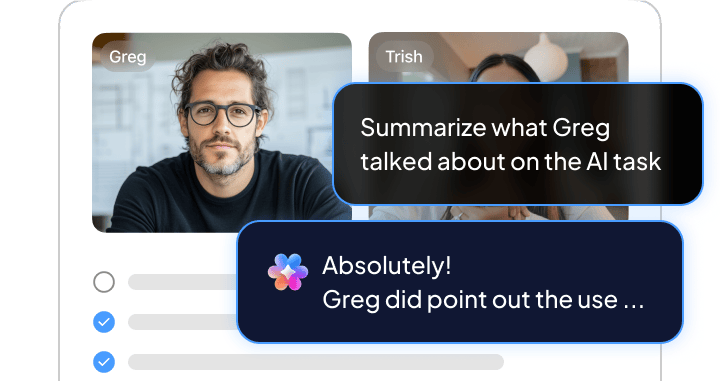
Smart Agenda Builder
Instantly create meeting agendas based on previous meetings, project status, and team priorities.
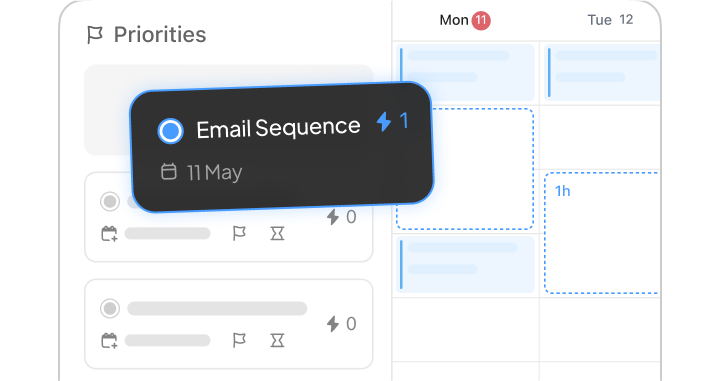
Enterprise AI Search & Ask
Cut the searching with answers from your Workspace and beyond
Connected Knowledge
Search across tasks, docs, chats, and tools to get instant answers with full context.
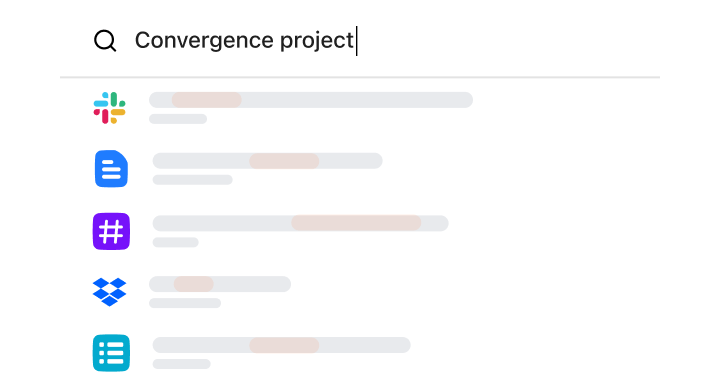
Org-Wide Search
One search gets you the right file, message, or task from across your Workspace, apps, and teams.
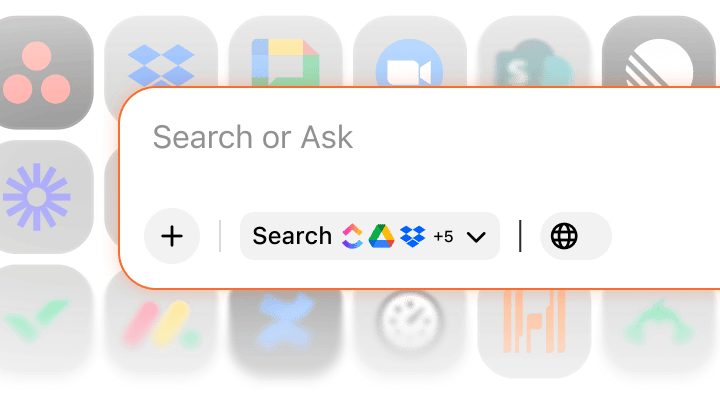
Every Premium LLM, One Brain
Use every major AI model in one place. ChatGPT, Claude, and much more.
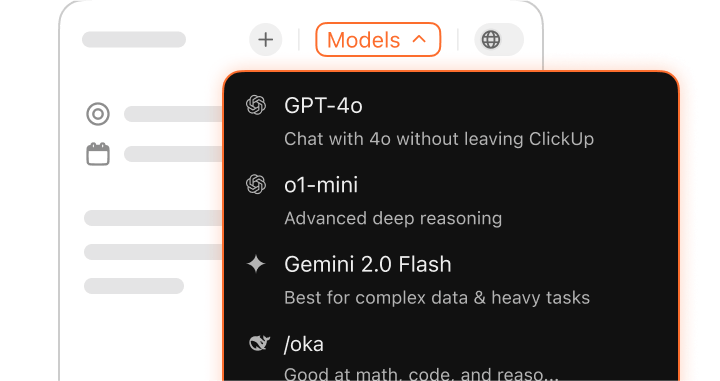
AI Creator
Create faster than ever with AI that turns ideas into visuals, words, and action
AI Image Generator
Generates on-brand visuals from your writing, no design skills or prompting required.
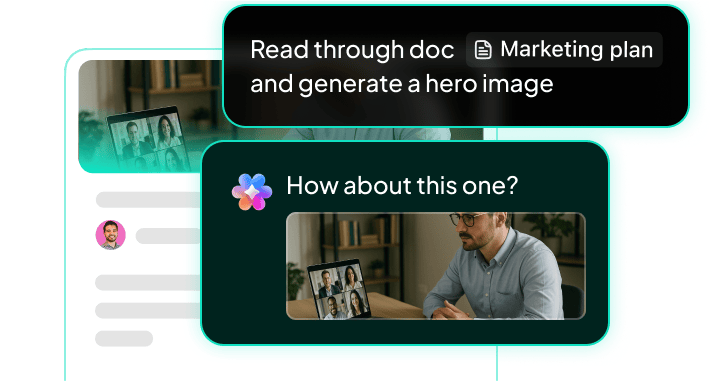
AI Doc Writer
Pulls action items from docs, chats, and meetings and turns them into fully built plans.
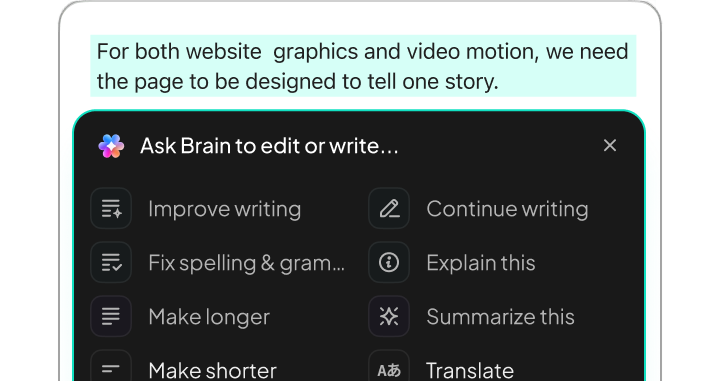
AI Task Builder
Creates detailed tasks from chats, docs, or meetings - no details missed.
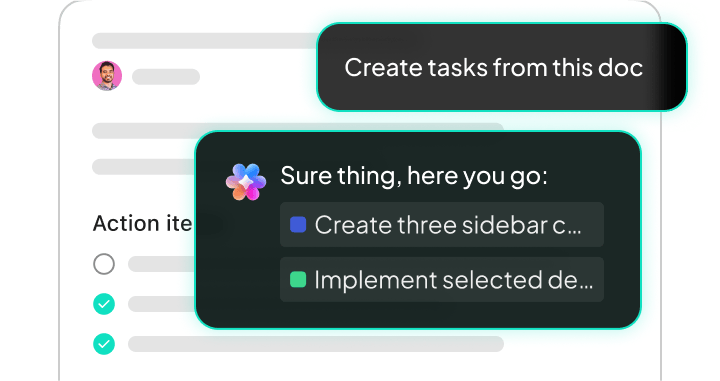
Enterprise-grade peace of mind
Your data stays secure and private with Brain Max.
Third-party AI providers never store or learn from your information.
Your AI-Powered Workspace